NEWS
Reducing Factory Downtime with Predictive Maintenance Tools
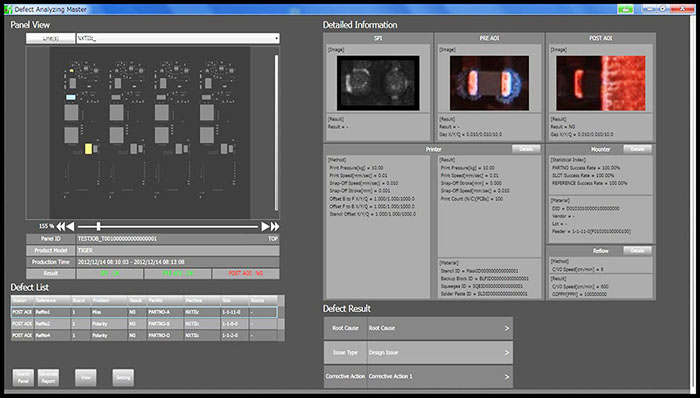
By Tony Picciola, Technical Services Manager, Fuji America Corporation
Whether it is reducing costs, optimizing utilization or enhancing the customer experience, Industry 4.0 can offer improvements in almost every aspect of the manufacturing process. However, there is one element that is a priority for nearly everyone: improved overall productivity. Overall productivity can be significantly improved through the use of Predictive Maintenance tools.
Predictive Maintenance
In any manufacturing Industry, most are familiar with “preventive” maintenance guidelines to avoid equipment failure. The goal is to reduce the likelihood of machine failures that result in factory downtime. Predictive maintenance starts as a philosophy that utilizes the production equipment’s operating conditions to make data-driven decisions.
With the rise of Industry 4.0, new data streams can be enabled with the use of inexpensive sensors to monitor how production equipment is performing in a variety of new ways. This information might include data collection of vibrations, temperature or power/amp fluctuations. With the use of this data and artificial intelligence (AI) software, we can now make predictions about future failures allowing operators to schedule corrective maintenance and prevent unexpected equipment failures. In SMT, we can define the objectives as improved overall productivity and improved maintenance efficiency. With the use of data collection and AI software, we can enter into a Predictive Maintenance operation and improve the overall machine uptime. By analyzing this data together, we can trigger a variety of behaviors, including taking corrective action if trends are found.
As production demands increase, many proactive tasks such as machine maintenance often fall behind. This reactive maintenance scheduling approach has been shown to have a negative impact to overall production line up time by 5-25 percent. With the use of predictive maintenance tools, production can continue to run until the data analytics provide a warning in advance. Maintenance can be planned around the production schedule creating an efficient process that also translates into fewer spare parts required to be kept on-hand due to the prediction of failures. This then reduces overall costs to maintain equipment as it can reduce the number of extra parts that may never be needed.
Improved Product Quality
Another direct benefit of implementing predictive maintenance is the improvement in the quality of the product that is being manufactured. Fuji carries prediction to the manufacturing process and creates a process analytics tool for defect monitoring. This software monitors historical processes to predict potential production variances at a very early stage by analyzing data from all machines throughout the process. This data includes monitoring the screen printing, SPI, pick-and-place, AOI, and reflow processes. All data is collected in one central location and analyzed for trends, equipment performance and process controls. The direct result of analyzing this data together allows Fuji to trigger a variety of behaviors, including taking corrective action if trends were found.
Possible corrective actions:
- Part placement locations can be adjusted, due to board warpage or solder paste misalignment. The end result is that the placement process was stabilized with the continuous monitoring of the data.
- Start a mask cleaning task.
- PCB panels that fail SPI inspection can be skipped before they enter the pick-and-place machine.
Within the pick-and-place process, the collection of machine feeder, nozzle and head performance data can be performed. This data can again be moved into a central data depository and software used to analyze it.
Instead of using “timed” preventive maintenance schedules for machine components such as feeders or nozzles, utilize a predictive schedule that monitors performance of these components and tracks the number of placements or picks.
By monitoring performance, trends can be identified immediately, and if necessary, the use of the feeder or nozzle can be disabled until a trained maintenance person performs a physical inspection.
By analyzing the data, a determination can be made of the cause of a trend. This might be from the component, its packaging or from an operator dropping components or feeders during the process. Either way, software can monitor trends that might occur as a result and provide specific feedback to the cause.
Setting up for Success
Companies can save time and money through the use of predictive maintenance tools by following a few guidelines. First, start small. Develop a pilot program that might start with the use of key vendor's software tools. This offers an early indication of how these changes can positively impact a business. Next, involve your IT early. They will need to assist with the data collection, either within the cloud or on premise servers/network ecosystem. Also, outline strategy with goals for predictive maintenance from the beginning. Every organization has its unique processes and finding the benefits for a particular company is critical.
Finally, as part of of the strategy, companies must decide if they will work with your production line vendors and utilize their software. In some cases, vendors are able to offer you a software solution that can go outside of their particular processes. MES software vendors are also evolving their software to assist with analyzing data collected from the entire line. This will continue to be the case as standards are established such as IPC-CFX, which allow more data to be exposed to anyone who wishes to collect it.
Conclusion
Industry 4.0 is continuing to evolve and there are many areas to be explored and many ways to improve business. The use of predictive maintenance tools is a way to get a company involved in Industry 4.0, as the many benefits and return on investment are clear. Companies should not be afraid to start a pilot and work with equipment suppliers to deploy what they already have available in the field. Once started, the benefits can be easily measured and quickly realized.
Please see published article in US Tech magazine